Forensic Analytics Methods And Techniques For Forensic Accounting: A Comprehensive Guide
Table of Contents
- Introduction
- What is Forensic Analytics?
- Key Methods of Forensic Analytics
- Data Mining and Pattern Recognition
- Anomaly Detection Techniques
- Link Analysis for Forensic Accounting
- Text Analytics in Forensic Accounting
- Tools and Software for Forensic Analytics
- Real-World Applications of Forensic Analytics
- Challenges and Limitations of Forensic Analytics
- Conclusion
Introduction
In today's digital age, forensic analytics methods and techniques for forensic accounting have become indispensable tools in the fight against financial fraud and misconduct. As businesses generate vast amounts of data daily, the need to analyze this data to uncover irregularities has grown exponentially. Forensic analytics combines advanced data analysis techniques with accounting expertise to detect and investigate financial crimes, ensuring transparency and accountability in financial reporting.
Forensic accounting is a specialized field that involves the application of accounting, auditing, and investigative skills to examine financial records. It plays a critical role in legal proceedings, regulatory compliance, and corporate governance. Forensic analytics, on the other hand, leverages technology and statistical methods to identify patterns, anomalies, and relationships within financial data. Together, these disciplines provide a robust framework for detecting fraud, preventing financial crimes, and supporting decision-making processes.
This article delves into the core methods and techniques of forensic analytics for forensic accounting, exploring their applications, tools, and challenges. Whether you're a financial professional, auditor, or business owner, understanding these techniques can empower you to safeguard your organization's financial integrity and ensure compliance with regulatory standards.
Read also:Peter Shamshiri Law School A Comprehensive Guide To Excellence In Legal Education
What is Forensic Analytics?
Forensic analytics refers to the application of data analysis techniques to uncover evidence of fraud, misconduct, or other irregularities in financial records. It involves the systematic examination of large datasets to identify patterns, anomalies, and relationships that may indicate fraudulent activities. Forensic analytics is a multidisciplinary field that combines elements of accounting, data science, and investigative techniques to provide actionable insights.
The primary goal of forensic analytics is to detect and prevent financial fraud by analyzing data in a way that reveals hidden patterns or irregularities. This can include identifying unusual transactions, detecting duplicate payments, or uncovering discrepancies in financial statements. Forensic analytics is particularly valuable in industries such as banking, insurance, and healthcare, where the volume of financial transactions is high and the risk of fraud is significant.
Key Characteristics of Forensic Analytics
- Data-driven approach to fraud detection
- Use of advanced statistical and analytical techniques
- Focus on uncovering hidden patterns and anomalies
- Integration with forensic accounting practices
- Support for legal and regulatory compliance
Key Methods of Forensic Analytics
Forensic analytics employs a variety of methods to analyze financial data and detect irregularities. These methods are designed to provide a comprehensive view of financial transactions and identify potential areas of concern. Below are some of the most widely used forensic analytics methods and techniques:
Data Mining
Data mining involves the extraction of patterns and insights from large datasets. In forensic analytics, data mining techniques are used to identify trends, correlations, and anomalies in financial data. This method is particularly useful for uncovering fraudulent activities that may not be immediately apparent through traditional auditing techniques.
Pattern Recognition
Pattern recognition focuses on identifying recurring patterns in financial data that may indicate fraudulent behavior. For example, irregular payment patterns or unusual transaction frequencies can signal potential fraud. Advanced algorithms and machine learning models are often used to enhance the accuracy of pattern recognition.
Anomaly Detection
Anomaly detection is a critical component of forensic analytics. It involves identifying outliers or deviations from expected patterns in financial data. These anomalies may indicate errors, fraud, or other irregularities that require further investigation. Statistical models and machine learning algorithms are commonly used to detect anomalies in large datasets.
Read also:Dyan Cannon A Legendary Actress And Her Remarkable Journey In Hollywood
Link Analysis
Link analysis examines relationships between entities, such as individuals, organizations, or transactions. This method is particularly useful for uncovering hidden connections or networks that may be involved in fraudulent activities. For example, link analysis can reveal connections between vendors and employees, which may indicate conflicts of interest or collusion.
Data Mining and Pattern Recognition
Data mining and pattern recognition are two of the most powerful tools in forensic analytics. These methods allow analysts to extract meaningful insights from large datasets and identify patterns that may indicate fraudulent activities.
How Data Mining Works
Data mining involves the use of algorithms to analyze large datasets and identify patterns or trends. In forensic analytics, data mining techniques are applied to financial data to uncover irregularities. For example, data mining can be used to identify duplicate payments, unusual transaction patterns, or discrepancies in financial statements.
Applications of Pattern Recognition
Pattern recognition is particularly useful for identifying recurring behaviors or trends that may indicate fraud. For instance, irregular payment patterns or unusual transaction frequencies can signal potential fraud. Advanced algorithms and machine learning models are often used to enhance the accuracy of pattern recognition.
Case Study: Detecting Fraud with Data Mining
A notable example of data mining in forensic analytics is its use in detecting fraudulent insurance claims. By analyzing historical claims data, analysts can identify patterns that are indicative of fraud, such as claims filed shortly after policy inception or claims with unusually high values.
Anomaly Detection Techniques
Anomaly detection is a cornerstone of forensic analytics, as it enables analysts to identify irregularities in financial data that may indicate fraud or errors. This section explores various anomaly detection techniques and their applications in forensic accounting.
Statistical Methods for Anomaly Detection
Statistical methods, such as standard deviation and z-scores, are commonly used to detect anomalies in financial data. These methods compare individual data points to the overall dataset to identify outliers. For example, a transaction amount that is significantly higher than the average may be flagged for further investigation.
Machine Learning for Anomaly Detection
Machine learning algorithms, such as clustering and neural networks, are increasingly being used for anomaly detection in forensic analytics. These algorithms can analyze large datasets and identify complex patterns that may not be apparent through traditional statistical methods.
Real-World Example: Anomaly Detection in Banking
In the banking industry, anomaly detection techniques are used to identify suspicious transactions, such as those involving large sums of money or transactions conducted in high-risk jurisdictions. These techniques help banks comply with anti-money laundering (AML) regulations and prevent financial crimes.
Link Analysis for Forensic Accounting
Link analysis is a powerful technique in forensic analytics that examines relationships between entities to uncover hidden connections or networks. This method is particularly useful for identifying collusion, conflicts of interest, or other forms of fraudulent behavior.
How Link Analysis Works
Link analysis involves mapping relationships between entities, such as individuals, organizations, or transactions. By visualizing these relationships, analysts can identify patterns that may indicate fraudulent activities. For example, link analysis can reveal connections between vendors and employees, which may indicate conflicts of interest or collusion.
Applications of Link Analysis
Link analysis is widely used in forensic accounting to investigate fraud, corruption, and other financial crimes. It is particularly effective in uncovering complex fraud schemes involving multiple parties or entities.
Case Study: Link Analysis in Corporate Fraud
A notable example of link analysis in forensic accounting is its use in investigating corporate fraud. By analyzing relationships between employees, vendors, and financial transactions, analysts can uncover hidden networks involved in fraudulent activities, such as kickbacks or embezzlement.
Text Analytics in Forensic Accounting
Text analytics involves the analysis of unstructured data, such as emails, invoices, and contracts, to uncover evidence of fraud or misconduct. This method is particularly useful for analyzing qualitative data that may not be captured in traditional financial records.
How Text Analytics Works
Text analytics uses natural language processing (NLP) techniques to analyze unstructured data and extract meaningful insights. For example, text analytics can be used to identify suspicious phrases or keywords in emails that may indicate fraudulent activities.
Applications of Text Analytics
Text analytics is widely used in forensic accounting to investigate fraud, corruption, and other financial crimes. It is particularly effective in analyzing qualitative data, such as emails, invoices, and contracts, to uncover evidence of misconduct.
Case Study: Text Analytics in Fraud Detection
A notable example of text analytics in forensic accounting is its use in detecting fraudulent invoices. By analyzing the text of invoices, analysts can identify discrepancies or irregularities that may indicate fraudulent activities, such as inflated amounts or duplicate entries.
Tools and Software for Forensic Analytics
Several tools and software platforms are available to support forensic analytics in forensic accounting. These tools provide advanced data analysis capabilities and are designed to help analysts uncover evidence of fraud or misconduct.
Popular Forensic Analytics Tools
- ACL Analytics
- IDEA
- Tableau
- Power BI
- Python and R for custom analytics
Features of Forensic Analytics Tools
- Data visualization capabilities
- Advanced statistical analysis
- Machine learning integration
- Support for large datasets
- Compliance with regulatory standards
Choosing the Right Tool
When selecting a forensic analytics tool, it is important to consider factors such as ease of use, scalability, and integration with existing systems. Additionally, the tool should support the specific needs of your organization, such as compliance with regulatory standards or the ability to analyze large datasets.
Real-World Applications of Forensic Analytics
Forensic analytics has a wide range of applications in various industries, from banking and insurance to healthcare and government. This section explores some of the most notable real-world applications of forensic analytics in forensic accounting.
Fraud Detection in Banking
Forensic analytics is widely used in the banking industry to detect fraudulent activities, such as money laundering, identity theft, and unauthorized transactions. By analyzing large datasets, banks can identify suspicious patterns and take proactive measures to prevent financial crimes.
Insurance Fraud Investigation
In the insurance industry, forensic analytics is used to investigate fraudulent claims. By analyzing historical claims data, insurers can identify patterns that are indicative of fraud, such as claims filed shortly after policy inception or claims with unusually high values.
Healthcare Fraud Detection
Forensic analytics is also used in the healthcare industry to detect fraudulent activities, such as billing fraud and overcharging. By analyzing medical records and billing data, healthcare organizations can identify discrepancies and ensure compliance with regulatory standards.
Challenges and Limitations of Forensic Analytics
While forensic analytics offers significant benefits, it also presents several challenges and limitations. Understanding these challenges is essential for organizations seeking to implement forensic analytics in their operations.
Data Quality Issues
One of the primary challenges of forensic analytics is ensuring the quality and accuracy of data. Poor data quality can lead to inaccurate results and false positives, undermining the effectiveness of forensic analytics.
Privacy Concerns
Forensic analytics often involves the analysis of sensitive data, such as personal information and financial records. This raises privacy concerns and requires organizations to implement robust data protection measures to ensure compliance with privacy regulations.
Cost and Resource Constraints
Implementing forensic analytics can be costly and resource-intensive, particularly for small and medium-sized organizations. This can limit the adoption of forensic analytics in some industries.
Conclusion
Forensic analytics methods and techniques for forensic accounting are essential tools for detecting and preventing financial fraud. By leveraging advanced data analysis techniques, organizations can uncover hidden patterns, anomalies, and relationships in financial data, ensuring transparency and accountability in financial reporting.
From data mining and pattern recognition to anomaly detection and link analysis, forensic analytics provides a comprehensive
Ritholtz Wealth Management Review: A Comprehensive Guide To Financial Success
Kok Digital Image Denoising In MATLAB Torrent: A Comprehensive Guide
Guardare Udemy The Absolute Beginners Guide To Cyber Security 2020 - Part 1: Your Ultimate Learning Journey
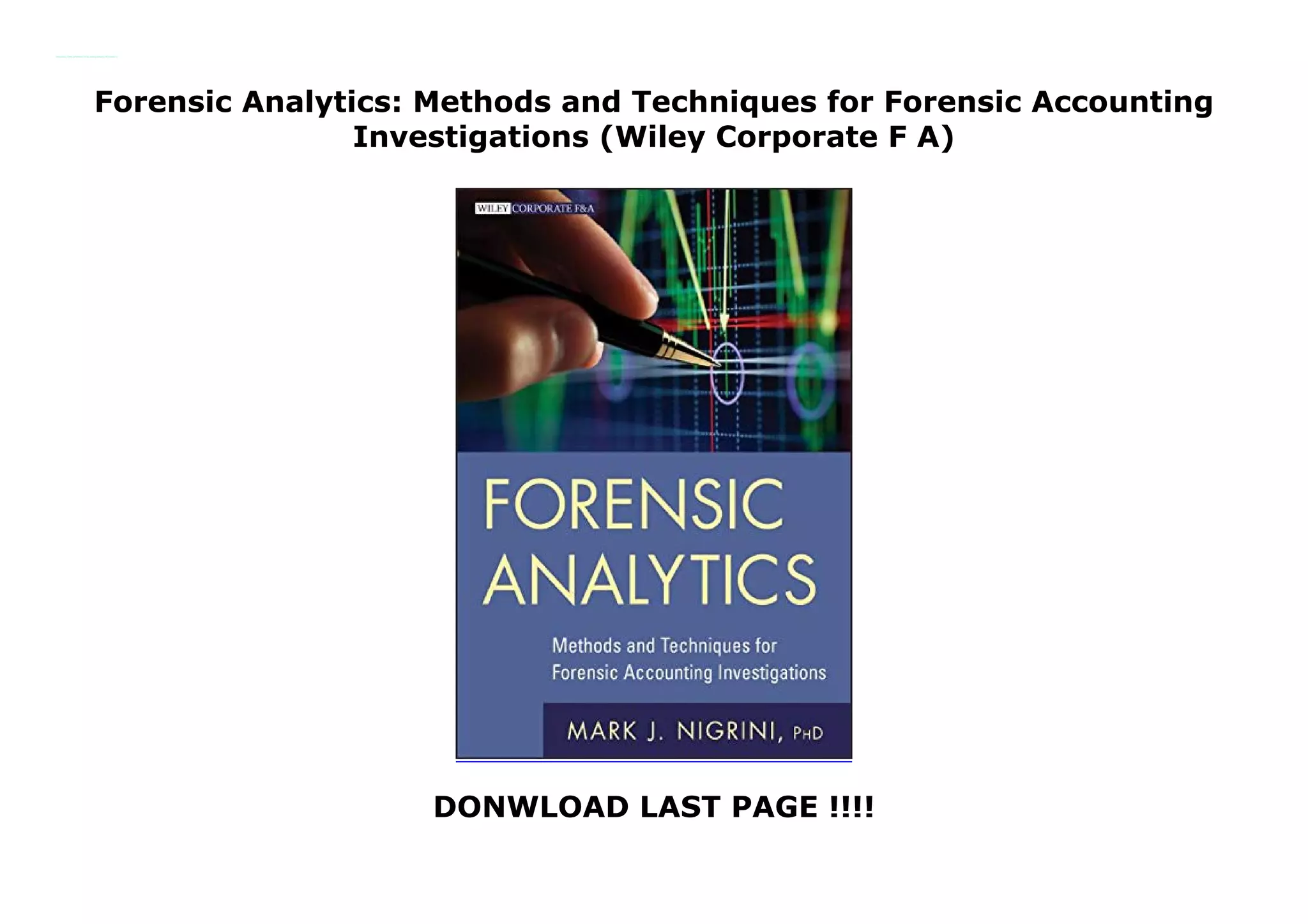
Forensic Analytics Methods and Techniques for Forensic Accounting
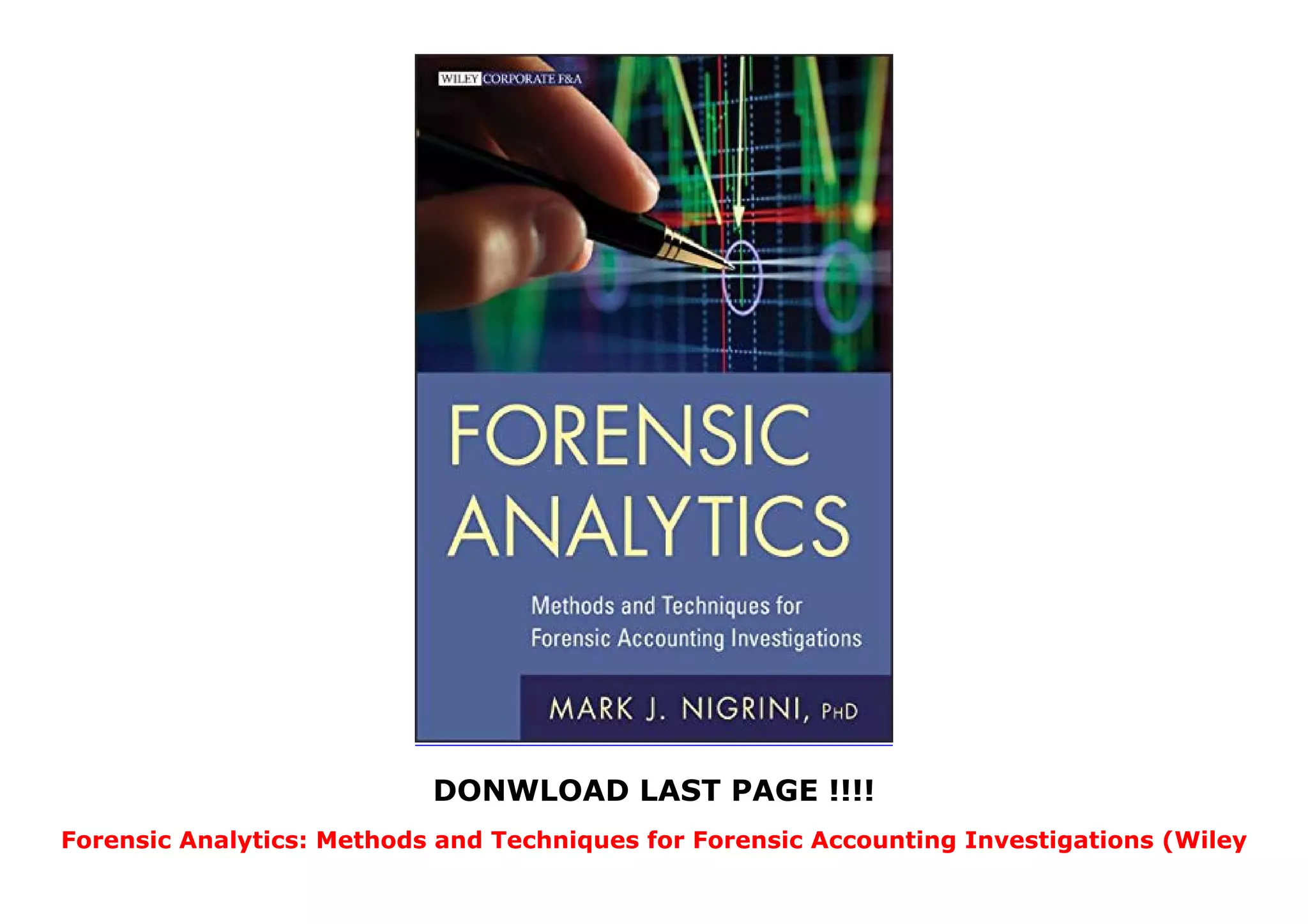
Forensic Analytics Methods and Techniques for Forensic Accounting